- Beyond The AI Horizon
- Posts
- AI Weekly Digest #30: AI Can Now Solve Algorithmic Problems better than Humans
AI Weekly Digest #30: AI Can Now Solve Algorithmic Problems better than Humans
GPT4, DeepMind FunSearch, Phi-2, Mistral MoE and Alibaba's Outfit Anyone

AI Weekly Digest #30: AI Can Now Solve Algorithmic Problems better than Humans
Hello, tech enthusiasts! This is Wassim Jouini and Welcome to my AI newsletter, where I bring you the latest advancements in Artificial Intelligence without the unnecessary hype.
Now let's dive into this week's news and explore the practical applications of AI across various sectors.
Main Headlines
What an AI week! I won’t be able to cover everything, but here’s what you need to keep in mind!
With the announcement of Gemini Ultra, Google best multimodal model, Google released benchmarks stating that Gemini was better than GPT-4! I did mention in my blog post that “the different methodologies used in testing and comparing Gemini with other models like GPT-4 make it challenging to draw definitive conclusions about its superiority”.
As expected OpenAI answered Google’s claims but releasing a new evaluation relying on the same metrics (including advanced prompting) to prove that GPT-4 is still the best on the market when it comes to text!
DeepMind continues to focus on solving major challenges with real life applications. Three notable achievements in the past:
AlphaGo: Supra human Go player beating the world champion in 2016!
AlphaFold: “The Most Important Achievement In AI — Ever” according to Forbes. AlphaFold was successfully applied in drug discovery to identify a hit molecule against a novel target in less than 30 days (instead of years).
Tokamak Control to enable stable nuclear fusion (read DeepMind’s blog post on this topic)!
This week, it announced FunSearch. An AI designed to solve complex algorithmic problem through iterative programming.
FunSearch has notably outperformed existing algorithms in challenging domains, such as the cap set problem in mathematics and the bin-packing problem in computer science. Its ability to generate concise, human-interpretable programs marks a step forward in the use of AI to uncover new knowledge and practical solutions in diverse fields.

Mistral Mixture of Experts (MoE) model was released a week ago and has demonstrated impressive performance, despite its smaller size. In academic benchmarks, it appears to be on par with GPT-3.5
What should you know about it?
It’s open source
Its academic performances are on par with GPT3.5. Based on my own early experiments, I can say that it’s at least as efficient as GPT3.5 on data extraction and classification (tested only on English though)
You can access it via together.ai at a 40% lower price compared to GPT3.5
It’s up to 2x time faster when relying on http://together.ai
Read this blog post (with code): to test Mistral MoE, and compare it with OpenAI models.
Disclamer: this newsletter IS NOT sponsored by http://together.ai
I am personally very excited about the recent unveiling of Microsoft's Phi-2.
This model represents a significant milestone in AI research, particularly in the context of the Phi program's objectives. Stemming from insights gained from GPT-4's analysis, the program aims to identify the minimal ingredients necessary for the emergence of 'sparks of intelligence' in AI systems.
The fact that today's AI models are scaling up in size—LLaMa with 70 billion parameters, GPT-3 with 175 billion, and GPT-4, rumored to be a mixture of 8 experts each with 200 billion parameters—makes Phi-2's approach all the more exciting.
Phi-2, with its 2.7 billion parameters, challenges the notion that larger is always better. It's fascinating to see that despite being significantly smaller, benchmarks have shown Phi-2 to be equivalent or even superior to larger models like LLaMA 2, which is a remarkable feat.
These results opens up new possibilities in AI research!
It raises the critical question: Can smaller models, when equipped with carefully curated, high-quality data, reach the same or potentially superior levels of performance?
Phi-2's achievements suggest that efficiency and compactness in AI models don't necessarily mean a compromise in capabilities. This could pave the way for more sustainable, accessible, and efficient AI solutions in the future, a prospect that I find incredibly promising
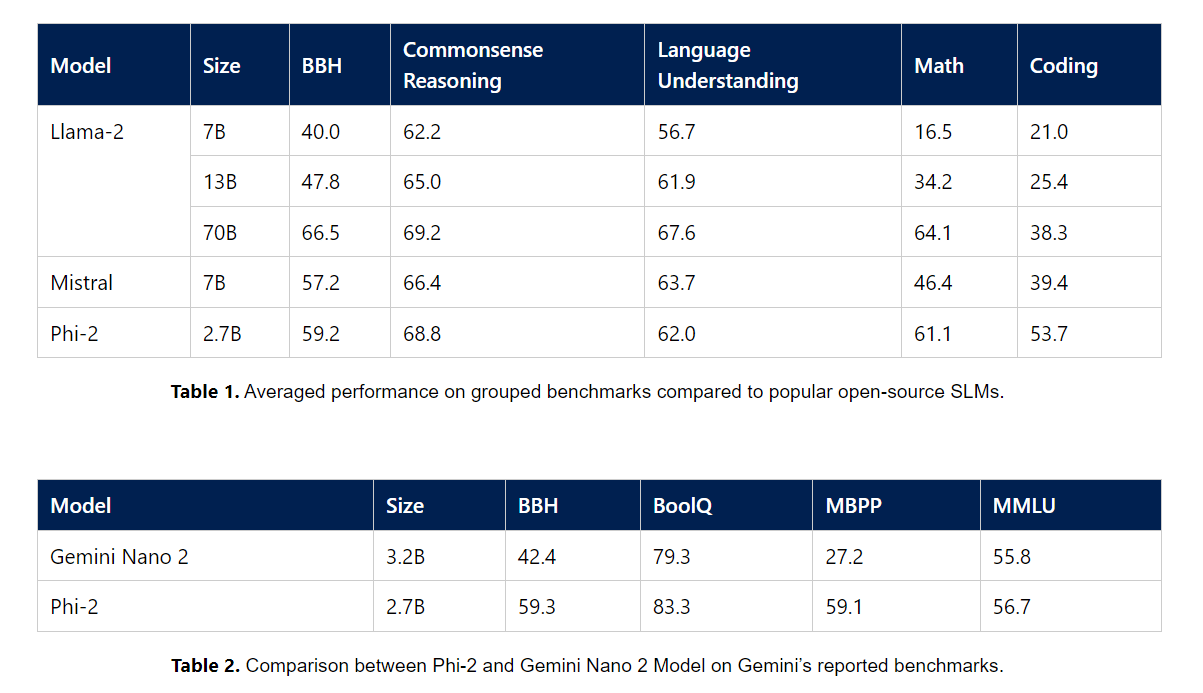
Phi2 is better than Meta’s LLaMA 2 70B parameters in math, coding, reasoning and so on despite the fact that it’s 26 times smaller!
Why do you need to care about this now?
The model is open source (limited to research for now, but probably not for long)
It’s size makes it fast and easy to deploy on a any infra provided it has a “decent” GPU
Fine-tuning it would be fast and cheap!
Phi2 is a great model to keep in mind for experimentation as you build your next AI systems for 2024!
Virtual try-on is a technology that allows users to digitally try on clothing without physically wearing them. This is achieved through software that superimposes garments onto images of people, giving a realistic impression of how the clothes would look when worn. The challenge in virtual try-on lies in accurately and realistically rendering the clothing on various body shapes and in different poses. Factors like the drape of the fabric, the interaction of the clothing with the body, and the maintenance of the garment's texture and color under different lighting conditions make it a complex task.
The research by the Institute for Intelligent Computing at Alibaba Group, titled "Outfit Anyone," represents a significant breakthrough in this area. It employs a two-stream conditional diffusion model to overcome the limitations of existing virtual try-on technologies. This advanced model allows for high-fidelity and detail-consistent rendering of clothing on people. It can adeptly handle garment deformation, making the results more lifelike and realistic.

Key features of "Outfit Anyone" include: The technology
adapts clothing to various body shapes and poses for realistic fitting,
creates initial try-on imagery without extensive data,
enhances clothing and skin texture details for improved realism,
offers versatility in styling from realistic to anime,
and integrates with motion video generation for dynamic outfit changes.
What does it mean? This means that we might be able to try out new outfits online using our photos or even try them on dynamically using a virtual mirror in shops.
This is it for Today!
Until next time, this is Wassim Jouini, signing off. See you in the next edition!
Have a great week and may AI always be on your side!